Ontvang 4x per jaar het laatste nieuws rond IT en dienstverlening.
Is data quality in your organisation up to par? Good, pleased to hear it! Then you can take the next step towards (even) better data quality! But there’s more to data quality than just measuring it. When it comes to really increasing that quality, measuring is just the beginning of the process: data cleansing. Data cleansing is the process of making data accurate and up-to-date, and it can be done in various ways. Business consultant Lorena Tol explains these ways and how to select the right “cleansing detergents” for your data problem.
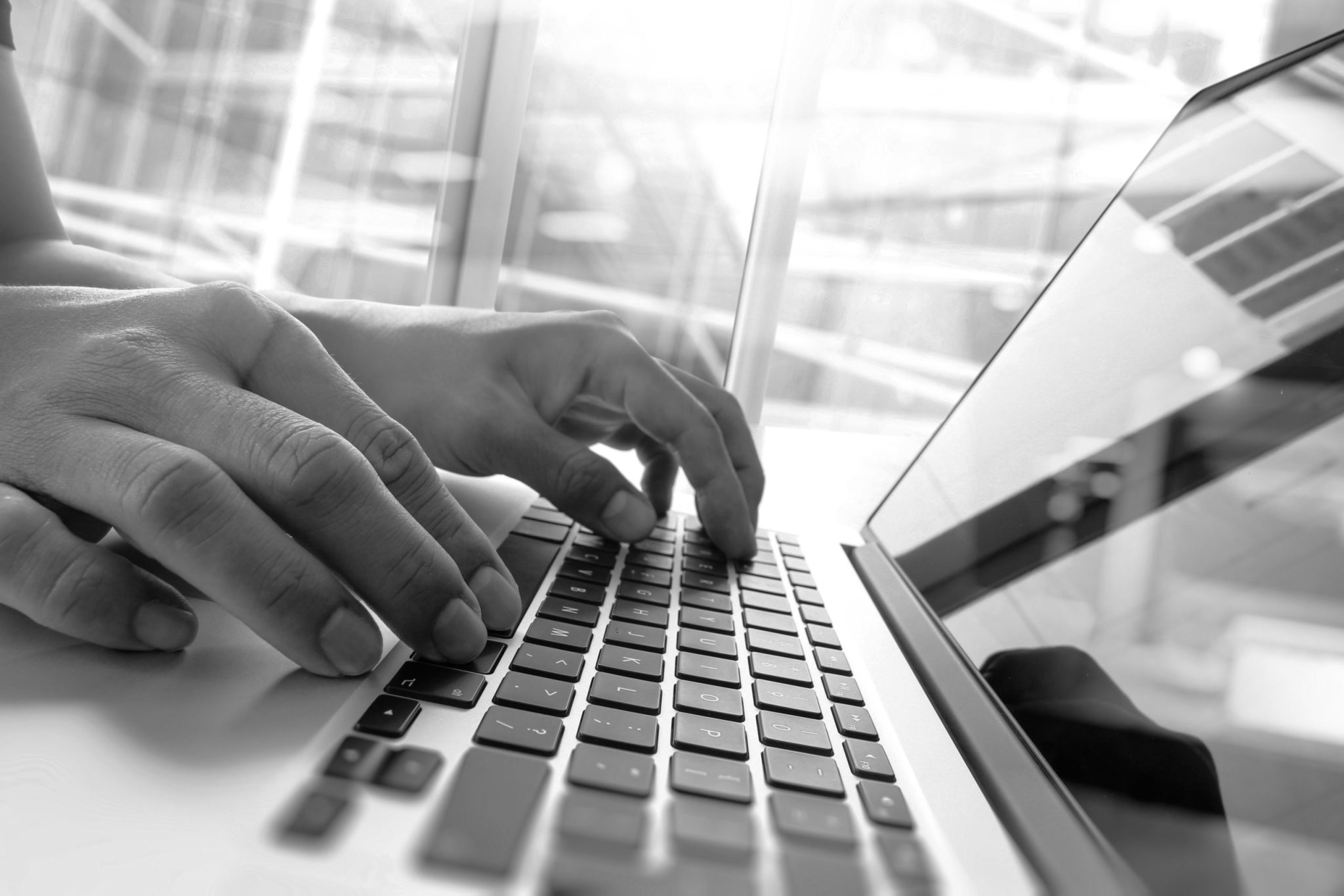
Data cleansing: what is it exactly?
Data cleansing is the process of finding and repairing missing or erroneous data, so that it meets the quality standards of the organisation and the regulatory authorities. In some organisations it is sometimes referred to as “data scrubbing”, a term that speaks for itself in this context. But how exactly do you clean or scrub your data? Well, it can be done in two ways: manually or automatically. Both methods come with advantages and disadvantages and each is better suited to specific data problems. Of course, a combination of both methods can also be used. Actually, in ITDS we see this combination as a popular solution in the market. So, what then are the characteristics of both these forms of data cleansing?
Manual versus automated data cleansing
Manual cleansing
In the case of manual cleansing, a data-quality analyst checks out the records individually. In so doing, he or she searches for the correct data in internal systems and archives, or trusted external sources (such as the Chamber of Commerce register). Sometimes it’s necessary to obtain or verify the required data by contacting the customer. Data-quality analysts can also be commissioned to actually repair data in the systems.
Automated data cleansing
The alternative to manual cleansing is to do it automatically, by using tooling to enrich and/or repair large numbers of records simultaneously. Two things are essential for successful automation: good preparation and the right tooling. Good preparation calls for a properly thought-through plan of action, in which clear requirements are drawn up. These include things like what the data should look like, where the necessary information must be obtained from, and under what circumstances the data will or won’t change. You also need the right infrastructure in which you can access the data in one place. Then you need tooling to carry out the necessary analyses and make the applicable corrections to the data in the systems.
The advantages and disadvantages at a glance
Manual cleansing
The manual cleansing of data is a time-consuming and cost-inefficient process. Moreover, the risk of errors increases when humans look up and adjust data manually in systems. This makes it essential that work agreements and clear control mechanisms for manual cleansing are properly documented. Otherwise, changing what might seem like a simple field, such as a Chamber of Commerce registration number, can easily take up a lot of time.
However, manual cleansing is unavoidable if looking up or improving data is likely to be so complex that the process cannot be covered by “rules” and is therefore impossible to automate. This will be the case, for example, if specific knowledge about a product and/or portfolio is required. It might also be the case that uploading data automatically is just not possible, or permitted.
Automated cleansing
Thanks to built-in control mechanisms, automated cleansing reduces the risk of human error. These mechanisms ensure that any errors in the cleansed data can be quickly detected and corrected. Furthermore, large numbers of data fields can be quickly cleansed in a standardised manner.
A disadvantage of automated cleansing is that the right tooling can be expensive. What’s more, not all data problems can be solved automatically, which means that manual analysis and repair will still be necessary.
An action plan for your data problem, in three steps
Every data problem is different, so how do you know which solution will solve yours? We use three steps for this.
- Assess the impact and priority of the data problem
This assessment will help to point you in the right direction in choosing a solution. If you don’t have a lot of time, for example, a manual solution might just take too long. - Check out where you can find the required data
Does it call for the specific knowledge of experienced specialists, for example, or is the data very easy to find in a standard place (such as in a certain archive folder)? The answer to this question will impact your options for using tooling. - Finally, determine how you want to process the enriched data in the systems
Can the data be uploaded to the source system as a batch? And will changing the data have the kind of direct impact on customers that means they will have to be approached individually?
Don’t keep banging your head against a brick wall
This might be a cliché, but that doesn’t make it any less true. Data cleansing is all about repairing existing data. However, it’s even more important to make sure that the data does not become corrupted again or that new data is entered incorrectly. For a structural solution it’s therefore crucial to trace the root cause of the data problem and tackle it. If you don’t, you’ll just keeping getting the same headache!
At ITDS we help our customers cleanse their data. Would you like to know more about our experiences with manual and automated data cleansing? Perhaps you’d like us to help you decide on the best data cleansing strategy for you, or how to tackle data problems at source? Just let us know! Contact Lorena at l.tol@itds.nl