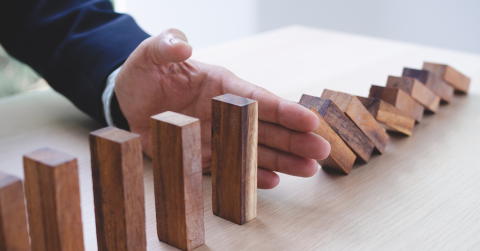
“Solutions, that’s the answer, not nice conceptual models”
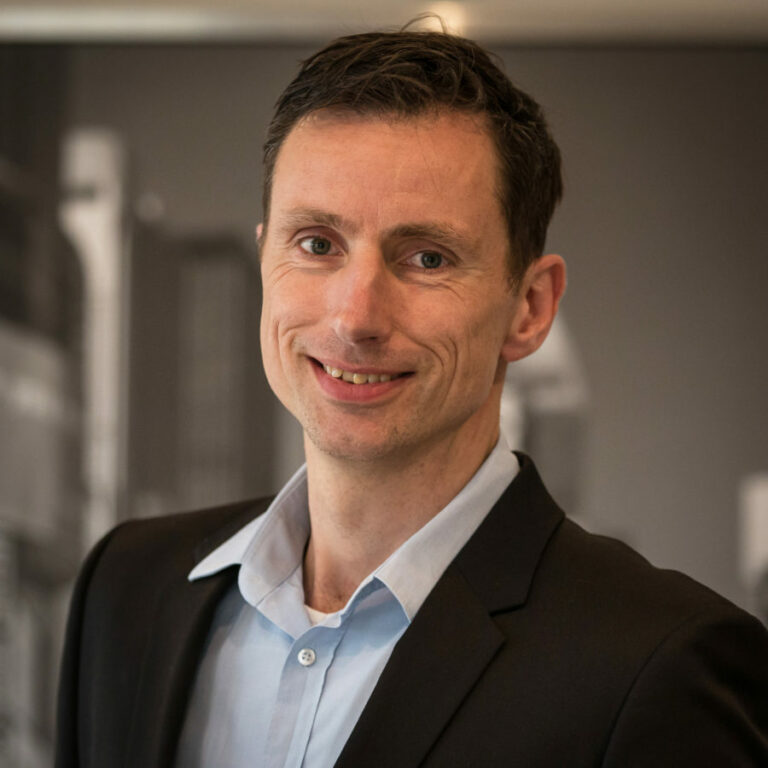
We don’t have a data-quality approach, we offer concrete solutions! That’s the first thing we’ll tell an insurer who is asking ITDS about this subject, which, on the face of it, is quite a tricky one. The second thing we’ll tell that insurer will probably be something along the lines of: “yes, of course we have an approach, but concrete solutions are so much more important.” And while there is no shortage of nice conceptual models, a CFO is expected to explain in understandable terms to management colleagues and supervisory staff how data quality works in practice. He or she must be able to identify strengths and weaknesses in the reporting chain. Then, regarding the latter, explain how bad they are and what’s being done about them. Fortunately, ITDS offers relevant solutions.
“PLENTY TO DO”
Several years ago DNB established that insurers are, by and large, at an early stage of developing and implementing a data quality policy, concluding that there’s still a lot of work to be done. As 2018 gets under way, it’s clear that many insurers are still struggling with data quality. A worst-case scenario will see an insurer facing an almost insurmountable mountain of documents, created solely to ensure compliance with DNB regulations and guidelines. Even better-case scenarios throw in elements in which there’s little or no cohesion. These can include the relationship between data lineage (the route that data takes from A to Z), the rules that apply to so-called EUCs (such as complex, interwoven Excel spreadsheets), risks in the process, the risk appetite and the procedure for “data issue resolution”. And then there’s the issue of why, as insurers, we consider it important that data quality needs to be up to scratch anyway, not to mention the associated culture, attitude and behaviour, from senior management all the way down to the shop floor. As DNB concluded, there’s more than enough to do!
INSIGHTS
There is no secret recipe for data quality, but there is such a thing as a down-to-earth perspective of how it should be interpreted. It starts with a visual insight into data flows within the reporting chain and the setting up of a number of data governance rules. This will give an insurer a foundation that can be further developed on the basis of practice. Shortcomings (issues) in data quality can be collated and made tangible in the visualisation of the reporting chain. The visual insights that this generates will serve as food for thought for the involved parties. Issues can be discussed, prioritised and solved. What’s important is that the vehicle that is data quality gains momentum and that it yields clear and demonstrable results. These include insights into what can and does go wrong, how this can be jointly rectified and how everyone will benefit from perceptions and figures that everyone can rely on.
Over time, the level of maturity will increase, by linking more characteristics to the issues that are encountered: the design, the results of second-line control testing, and all that can go wrong in the real world. Incidentally, the importance of the latter shouldn’t be underestimated. What that final green tick for a control phase doesn’t show, is the sheer misery that often has to be contended with in getting certain data correct.
It all starts then with the basics, the visualisation and some ground rules. From here, we can flesh out all the elements of the data framework in a way that’s a good fit with the insurer. Of course, in doing so you’ll need to decide how far you want to go in working out the lineage (everything on an individual data level, really?), can you rely on the demonstrably correct functioning of the EUCs, and how can you make the quality of the data immediately discernable? Then you’ll need to establish the most convenient way to explain the relationships between risks, issues and risk appetite. Solutions, that’s the answer, not nice conceptual models.